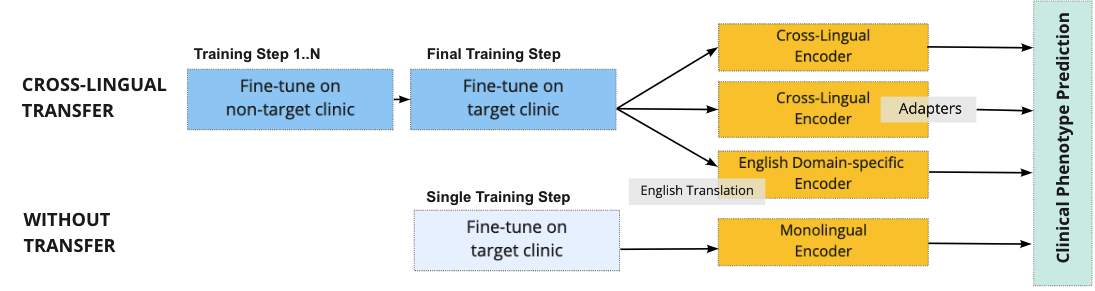
Clinical phenotyping enables the automatic extraction of clinical conditions from patient records, which can be beneficial to doctors and clinics worldwide. It is especially beneficial for the automatic diagnosis of diseases that up to now do not occur often at a geographic location as at another or when the population e.g. like in Greece is relatively small. Here, we investigated cross-lingual knowledge transfer strategies to execute this task for clinics that do not use the English language and have a small amount of in-domain data available. We evaluated these strategies for a Greek and a Spanish clinic leveraging clinical notes from different clinical domains such as cardiology, oncology and the ICU. We examined two different cross-lingual knowledge transfer strategies and found that both perform especially well for classifying rare phenotypes and we advise on which method to prefer in which situation. Our results show that using multilingual data overall improves clinical phenotyping models and can compensate for small datasets.
Article link: http://www.lrec-conf.org/proceedings/lrec2022/pdf/2022.lrec-1.95.pdf
Poster: https://s3.eu-west-2.wasabisys.com/lrec2022/posters/257.pdf
Video: https://s3.eu-west-2.wasabisys.com/lrec2022/posters/257.mp4